In this blog post I want to talk about large language models (LLMs) – a type of artificial intelligence (AI) designed to generate human-like text in response to a verbal query or prompt, such as OpenAI’s ChatGPT and Google’s Bard.
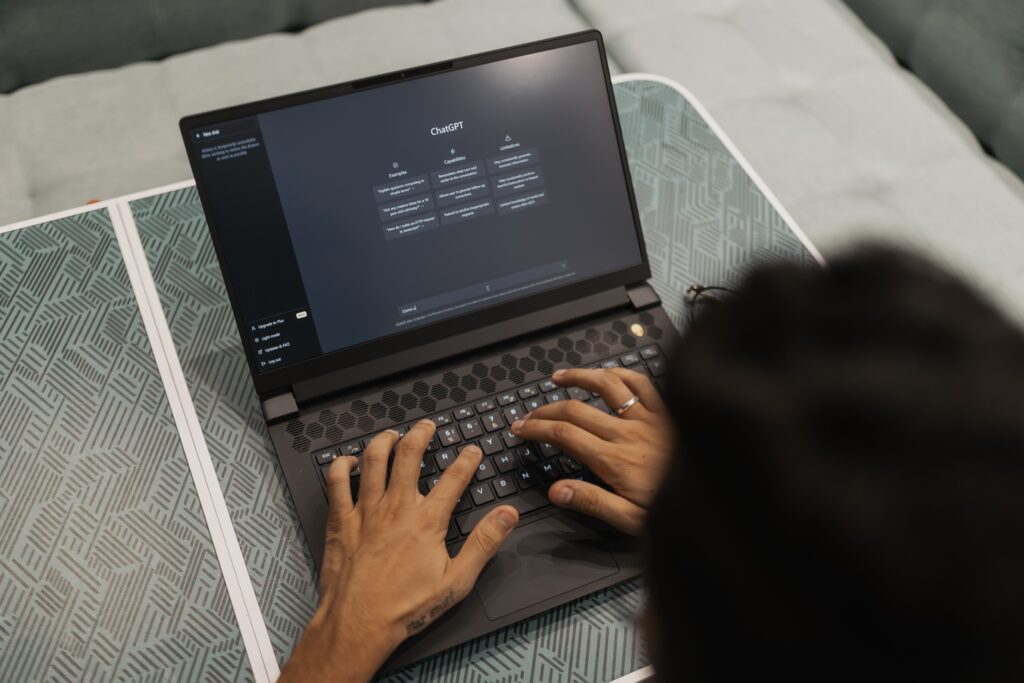
These models have the potential to change the way we work, whether that’s how we write our emails, summarise documents, create reports or analyse data. Whether or not your organisation has a policy on if and how to use LLMs, it’s almost certain people in your organisation are already using them one way or another.
We are not even close to understanding the full impact AI will have on our society. Right now however, the spotlight is shining on LLMs. For that reason, I want to take some time to outline key facts any organisation, but especially those in the public sector, must consider. Before implementing anything, it is vital to understand how these technologies work, what risks they introduce to an organisation, how to go about mitigating those risks, and implementing them safely.
If you are considering building a business case for an AI project within the public sector you can read our full guide here.
Regulation and AI
As technologists, it’s hard not to be excited by these new tools. But coupled with that excitement is our responsibility to make sure we use them safely. After all, it was as recently as March that some of the world’s leading technologists signed a letter calling for a pause on AI development1.
Right now there’s a lack of regulation around AI, with nations taking a number of different approaches2. At the time of writing, Britain is simply applying existing regulations to AI systems, while the EU has begun categorising different uses of AI by degrees of risk. This has led to some outright bans, for example in subliminal advertising.
The core of government concerns are around accountability, privacy, bias and even intellectual property rights. It has become a regular occurrence to find new news stories about LLMs plagiarising writers3 and artists4, and even fabricating non-existent lawsuits5.
In March, the Department for Science, Innovation & Technology (DSIT) published an excellent white paper, A pro-innovation approach to AI regulation6, outlining 5 values that every implementation of AI should embody:
- safety, security and robustness
- fairness
- transparency and explainability
- accountability and governance
- contestability and redress
Let’s look at some of the things public sector organisations should consider when approaching these values.
1. Safety, security and robustness
Perhaps the main consideration right now is that the readily-available LLMs are proprietary technology run by private companies. No online service is completely safe. In a fast-moving technology landscape, being first to release a product to market can be a significant advantage – perhaps to the tune of billions of dollars.
There have already been examples of data breaches with LLMs7. These have involved selling stolen credentials and users having their chat histories exposed to other users. If, say, you’re asking for a list of the best places to eat in London then that may not be such a big deal. But where LLMs are used in the workplace, these breaches pose more serious questions. Government demands a higher standard and greater certainty.
It’s also worth bearing in mind that any information you share with an AI is going to a third-party server, which currently means outside of the UK. Entering sensitive information – especially personally identifiable information, may not only be against your organisation’s policy – it may be illegal. When it comes to making good decisions about what information to enter into an LLM, skew cautious.
It’s also important to understand how the data entered into LLMs is used. In the case of ChatGPT, unless you actively opt out8, information you share is stored to train future models. Even if you opt out, your conversation is held for 30 days and reviewed.
Companies like Amazon, Apple, and JPMorgan Chase & Co have banned their employees from using third party LLMs for reasons of data privacy9. Recently, Samsung10 bought in a company wide ban due to sensitive source code being leaked by employees.
The UK government has very strict policies around data being stored onshore. ChatGPT is owned by Open AI, an American company and does not have data servers in the EU or UK. At the moment, there’s no national LLM in the UK11. That means your data and personal information will be transferred to servers in the States. It’s vital to consider those implications when deciding what information to share with LLMs.
2. Fairness
Large language models reflect the data set they’re trained on – the web. LLMs are trained by scraping data from hundreds of thousands of websites12 and their outputs sometimes reflect the errors, biases and other imperfections that exist in that data13.
If you ask ChatGPT about biases in its data, it will likely respond along these lines:
“Yes, language models can have biases, because the training data reflects the biases present in society from which that data was collected. For example, gender and racial biases are prevalent in many real-world datasets, and if a language model is trained on that, it can perpetuate and amplify these biases in its predictions.”
In this case, spot on. Like addressing any kind of bias, there’s no quick fix. And unfortunately, there’s nothing you can do to change your text inputs to be sure of removing bias. As convincing as its answer is, ChatGPT doesn’t understand bias as a concept, and doesn’t make choices about which data it uses to formulate text.
In one example, when ChatGPT was asked to write a python function to see if someone would make a good scientist based on race and gender, it returned clauses to check if the candidate was white and male14. To be sure, the prompt was designed to expose bias, but expose bias it did.
The best you can do is to be aware of biases at all times, and scrutinise both the inputs and outputs. This is the first of several reasons we’ll come onto that you should be extremely cautious about using LLM outputs verbatim.
3. Transparency and explainability
Language models are extremely complex. Under the bonnet, they are neural networks which emulate organic brains. And much like the brains they’re modelled on, the inner workings of neural networks are not well understood.
This is another reason to be extremely cautious of using LLM outputs verbatim. It’s practically impossible to say how the model arrived at them. This will improve. Tools are being developed specifically to peer into their inner workings and privately trained models will require disclosure of the data used to develop them. For now though, LLMs remain a black-box technology.
LLMs are sometimes positioned as the sum total of human knowledge. But it’s perhaps more realistic to view them as the aggregate of human data – knowledge and misinformation alike. When using AI to create text, remember that they are incapable of original thought or ideation. The best you can hope for is plausibly derivative. In many use cases, that may be enough – if you can verify that the information is original and correct.
The outputs of LLMs can look extremely convincing at face value. But cracks can quite quickly appear when you probe the detail of subject matter you’re more familiar with. What’s important to remember is that those same potential cracks exist for subject matter you’re less familiar with. Therefore, it’s important to scrutinise the outputs of LLMs with subject matter experts before they’re put to any serious use.
4. Accountability and governance
An important part of government is accountability and a fundamental component of society is our democratic process. We elect individuals to represent us and our interests. It’s their job to make difficult decisions and act in our best interests. As a society we must be able to hear meaningful explanations for these decisions.
Outsourcing this responsibility to AI carries the risk that decisions are not clearly expressed or easily understood, or that the reasoning behind them isn’t clear.
There is also growing awareness of AI’s potential to be used to cause harm – for example by propagating misinformation via social media. The public sector will need to find a way forward that remains in step with public opinion on the benefits and risks of AI. While society as a whole adapts to AI, caution is recommended.
Where AI is used, it should be made clear to end users that this is the case, with some clear information on how it’s used, along with an explanation of any limitations and risks in that context.
See the research from Centre for Data Ethics and Innovation on AI governance15 for some good insights in this area.
5. Contestability and redress
Returning to the DSIT white paper, A pro-innovation approach to AI regulation6, it argues that it should be possible to contest a harmful decision or outcome generated by AI.
Here, we’re going beyond LLMs specifically and into AI more broadly. These potential use cases go beyond “safer” uses, such as to inform research or early stage draft content, but rather inform decision-making with real world implications. In these cases, people potentially affected by those decisions have a right to understand how AI is being put to use.
Tax is a good example of a sensible third way for using AI to inform decision-making rather than deferring to it. AI tends to be better suited to narrow, rigidly-definable tasks than analysing softer situations and problems. One strength of artificial intelligence is its ability to process large amounts of data to identify, for example, outliers and other anomalies. In the case of tax, that may be irregularities in the data that point to potentially-fraudulent behaviour. These can then be flagged up for human scrutiny and final decision-making.
At Made Tech, we regularly deploy AI tools, the workings of which are understood and which can be clearly explained. We use these to help clients with activities like topic modelling, sentiment analysis and predictive forecasting.
Ultimately, government deals with real people with real problems that algorithms can’t hope to fully grasp. Before deferring decisions to AI, it’s important to grasp what tasks AIs are adept at handling – and which they aren’t.
Ethical considerations
In some cases, the “intelligence” behind language models funnels down to a human being reading and labelling your inputs. This person could theoretically be anyone anywhere in the world.
Using humans to sort and label data isn’t a new phenomenon. The Mechanical Turk has long been a service offered by Amazon. This is a service that lets you outsource tasks to people around the world.
“The computer has a task that is easy for a human but extraordinarily hard for the computer,” Amazon founder Jeff Bezos explained to the New York Times back in 200716. “So instead of calling a computer service to perform the function, it calls a human…”
LLMs are taking a similar approach, and in some cases labour is outsourced to low-paid workers in developing countries. Sometimes this involves exploitative working practices, as reported in articles by Time17, Noema18 and MIT Technology Review19.
It’s also important to understand that LLMs themselves don’t have a concept of right or wrong. Here again, it’s useful to understand how LLMs operate. When they generate text, they’re not making smart choices – they’re simply weighing up the probability of what word is most likely to come next in a string of text based on the wealth of information published online.
As I am sure you can imagine, consuming the entire internet is going to have a serious impact on anybody’s ability to distinguish reality from fiction. LLMs are no exception. LLMs are so well known for presenting completely made up information the phenomenon even has its own name: LLM hallucinations. In one example, a lawyer used case law and precedent generated by LLMs in court filings, only to find his citations didn’t exist20. In another incident, a radio host is now suing OpenAI for defamation based on false information generated by ChatGPT.21
As I mentioned previously, plagiarism is also a consideration. LLMs don’t create original content. When we asked ChatGPT to “compose” a zen koan, it offered up “what is the sound of one hand clapping?” – the most well-known koan in its most common English translation. It doesn’t matter what verb you issue as a command – all ChatGPT can do is assemble sentences hashed together based on things already written.
At the very least, it’s highly recommended to run any outputs from an LLM through a plagiarism checker. Grammarly has a free plagiarism checking tool you can use in a browser.
It’s worth noting that this extends beyond blog posts and academic papers – it’s also true for code. This can risk introducing vulnerabilities and other bugs into your software.22
This also extends beyond LLMs to image-generation AIs. We’re beginning to see class-action lawsuits against AI image generators on the premise that they can’t help but plagiarise the source material on which they’re trained, with artists’ work going uncredited23. Few if any precedents around AI and copyright law exist, so again, caution is needed.
Weighing up the options
It’s worth repeating here that we’re talking about one kind of AI which, at the moment, is largely available through third-party companies.
Broadly, though there are so many unknowns (including unknown unknowns) about how AI will affect society, what we can be certain of is that it will – and significantly. But right now, whether to use third-party LLMs – and how – is a question every organisation needs to engage with, and there’s no one right answer.
For organisations experimenting with the technology, there are 2 essential considerations. Be informed. Be careful. The conclusion that, in their current form, third-party LLMs are not suitable for public sector use is a perfectly valid conclusion. Of course this is not to say that AI doesn’t have huge scope to transform the way government is done, as the government has itself identified with an initial £100 million investment into a taskforce to help the UK develop next-generation safe AI.
But if your organisation wants to use LLMs, there are some less risky ways that could produce benefits, if you’re judicious about what you put in, and how you use what you get out. For example, if you’re creating non-sensitive copy for the public domain, you could try asking ChatGPT to rephrase longer sentences into more accessible English. But you should take the extra steps of checking that the output hasn’t changed its meaning, while also running the finished product through a plagiarism checker.
Another possible use is content ideation. If you’re drafting a blog post, report or white paper, you could ask an LLM to suggest some topic areas, and high-level talking points, while remaining vigilant of not inputting sensitive information. Bear in mind it’s not going to suggest anything original, but it may help you to not miss out on points you should cover.
The Cabinet Office has put together a helpful guide on using generative AI25. With guidance on what to avoid and where it can be useful, there are many ways the public sector can start to benefit from this technology in a safe and responsible way. From research to summarising information, technology can support the work civil servants do every day.
Large language models are impressive tools. But anyone who is considering using them must know the limitations, do their due diligence, and understand that using LLMs carries risk for both individuals and organisations. We have to be vigilant when it comes to the public sector and people’s personal and private data. If you do choose to use LLMs, be selfish: get more out than you put in.
If you’d like to talk about how your organisation can best engage with AI technology, feel free to contact me or our Head of Data Jim Stamp via our data page.
End notes
- Pausing AI Developments Isn’t Enough. We Need to Shut it All Down (Time)
- How to worry wisely about artificial intelligence (The Economist)
- Google Bard Plagiarized Our Article, Then Apologized When Caught (Tom’s Hardware)
- Artists Are Suing Over Stable Diffusion Stealing Their Work for AI Art (Motherboard)
- OpenAI faces defamation suit after ChatGPT completely fabricated another lawsuit
- A pro-innovation approach to AI regulation (Department for Science, Innovation and Technology and Office for Artificial Intelligence)
- Massive Leak Of ChatGPT Credentials: Over 100,000 Accounts Affected (Search Engine Journal)
- Data usage for consumer services FAQ (OpenAI)
- Amazon, Apple, and 12 other major companies that have restricted employees from using ChatGPT (Business Insider)
- Lessons learned from ChatGPT’s Samsung leak (Cybernews)
- Could UK build a national large language AI model to power tools like ChatGPT? (Tech Monitor)
- Where Does ChatGPT Get Its Data From? (Tooabstractive)
- Uncovering The Different Types Of ChatGPT Bias (Forbes)
- ChatGPT could be used for good, but like many other AI models, it’s rife with racist and discriminatory bias (Business Insider)
- CDEI publishes research on AI governance (Centre for Data Ethics and Innovation)
- Artificial Intelligence, With Help From the Humans (The New York Times)
- Exclusive: OpenAI Used Kenyan Workers on Less Than $2 Per Hour to Make ChatGPT Less Toxic (Time)
- The Exploited Labor Behind Artificial Intelligence (Noema)
- How the AI industry profits from catastrophe (MIT Technology Review)
- Here’s What Happens When Your Lawyer Uses ChatGPT (New York Times)
- OpenAI faces defamation suit after ChatGPT completely fabricated another lawsuit (Ars Technica)
- The Dark Side of Large Language Models (Hidden Layer)
- Is A.I. Art Stealing from Artists? (New Yorker)
- Initial £100 million for expert taskforce to help UK build and adopt next generation of safe AI (Department for Science, Innovation and Technology, Prime Minister’s Office, 10 Downing Street)
- Guidance to civil servants on use of generative AI (Cabinet Office)